Leveraging Powerful
OpenSource Technology
Creating a strong foundation for the SigmaX Nexus and Chronicle Family of Stackable Accelerator Products
We proudly leverage Apache Arrow and Apache Pulsar as the foundational technologies of our products. Apache Arrow enhances our capabilities with its advanced columnar memory format, enabling rapid and efficient data analysis across various platforms. Concurrently, Apache Pulsar strengthens our infrastructure with robust, scalable solutions that guarantee low latency and high throughput for real-time data needs.
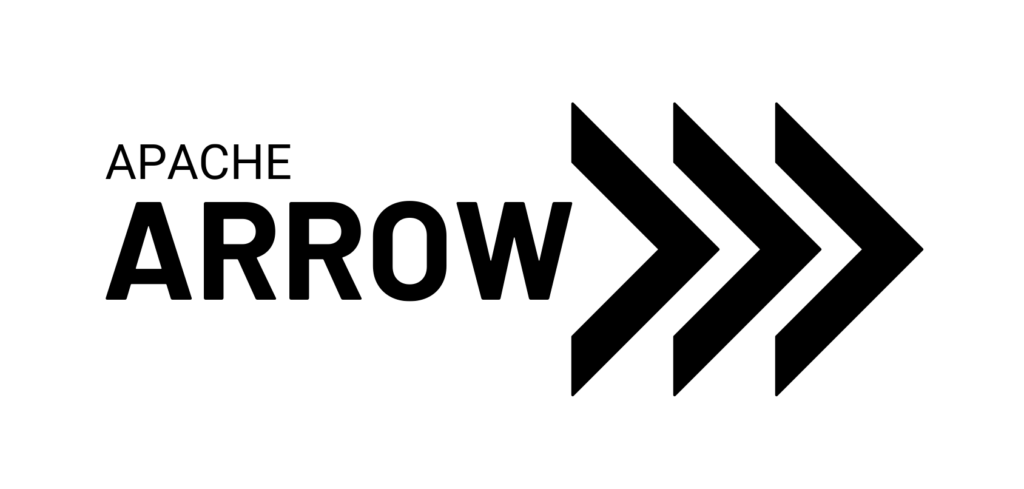
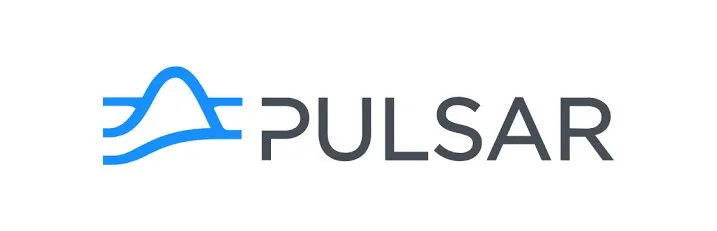
Elevating Data Processing with Apache Arrow
Our product harnesses the power of Apache Arrow to redefine data processing efficiency. This advanced in-memory data format is the engine behind our swift analytics and data-intensive operations, offering a competitive edge in the marketplace.
Strategic Advantage : Arrow’s columnar structure translates to superior speed and efficiency in data handling, aligning with the technical vision for agile and responsive systems.
Operational Value : By enabling faster decision-making and bolstering AI and ML initiatives, our product positions the company as a leader in innovation and customer satisfaction.
Financial Impact : The efficiency gains from using Arrow not only mean cost savings in data processing but also optimize resource allocation for greater ROI on data investments.
Redefining Data Transport with Apache Arrow Flight
Apache Arrow Flight is a game-changer for networked data transport, ensuring that massive datasets move quickly and securely across the network, driving real-time business insights.
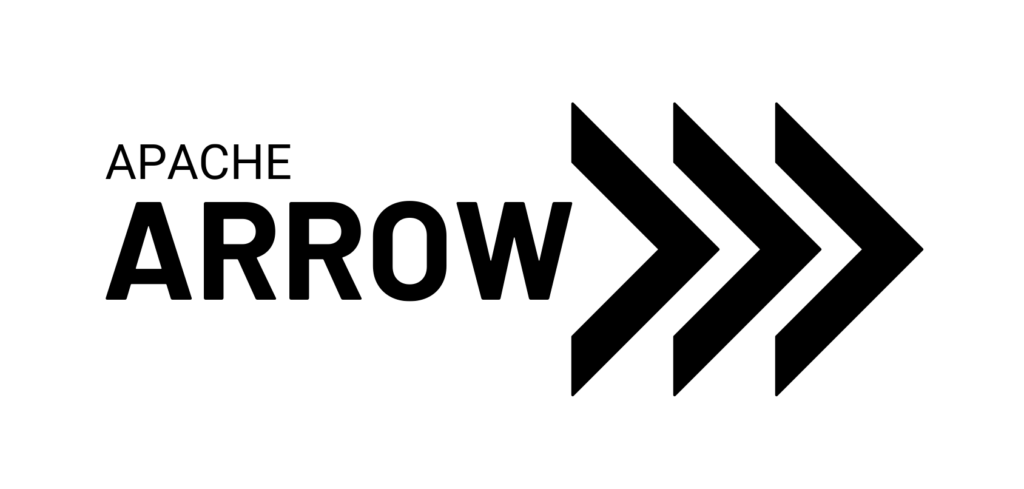
Strategic Advantage : With Arrow Flight’s high-speed data movement capabilities, we ensure that your technology infrastructure is future-proof and scalable.
Operational Value : This seamless data transfer empowers your team to unlock new business opportunities and elevate customer experiences through timely insights.
Financial Impact : Efficient data transport translates to reduced network overhead, leading to significant cost efficiencies and a leaner data infrastructure.
Streamlining Real-Time Messaging with
Apache Pulsar
Apache Pulsar is at the core of our messaging and streaming services, providing unparalleled throughput and reliability for real-time data needs, critical for today’s digital economy.
Strategic Advantage : Pulsar’s architectural flexibility and performance metrics support the most demanding enterprise applications, ensuring robust and uninterrupted service delivery.
Operational Value : Leveraging Pulsar’s capabilities means your organization can reliably process and analyze data streams, translating to enhanced agility and market responsiveness.
Financial Impact : The scalability and efficiency of Pulsar contribute directly to cost control by optimizing cloud resource utilization and reducing operational risks.
Harnessing Distributed SQL Queries with Apache Presto
With Apache Presto, our distributed SQL query engine, we bring the power of big data analytics to your fingertips, enabling fast, intelligent decision-making.
Strategic Advantage : Presto’s ability to perform federated queries across diverse data sets allows for unmatched flexibility in data strategy and technology innovation.
Operational Value : Instant access to insights from big data analytics can drive strategy, improve customer engagement, and foster a data-driven culture.
Financial Impact : By enhancing Presto with our product, we ensure the acceleration of time-to-insight, leading to better operational decisions and ultimately a healthier bottom line through data-driven optimization.
SigmaX’s Commitment to Apache Open Source Software
Our commitment to the Apache community is deep-rooted. We actively contribute to core improvements and drive innovation within these projects. Our team has not only achieved several world-firsts using these technologies but has also played a pivotal role in Apache’s New Project Leadership initiatives, furthering our leadership in the industry and setting new benchmarks for technological advancement.
World Firsts at SigmaXai
We create revolutionary software to transform how models consume data.
Apache Core Improvements
- Page-Aligned Buffers for FPGA/GPU processing Type Handlers
- PR’s for Pulsar, Arrow, Arrow Flight
- Improvements to Apache Fletcher for expanded Datasets
Apache New Project Leadership
- Apache Bolson Messaging Saturation Test Harness
- Apache Illex Random At-Scale Data Generator
- Apache Dive defining low-power device pre-processing and query of data
Featured Resources
Pulsar vs Kafka: The Future of Data Streaming in Enterprise Systems
When it comes to handling big data, particularly within enterprise applications, the debate often comes down to Apache Pulsar and Apache Kafka. Both systems serve as a backbone for messaging and stream processing, but the
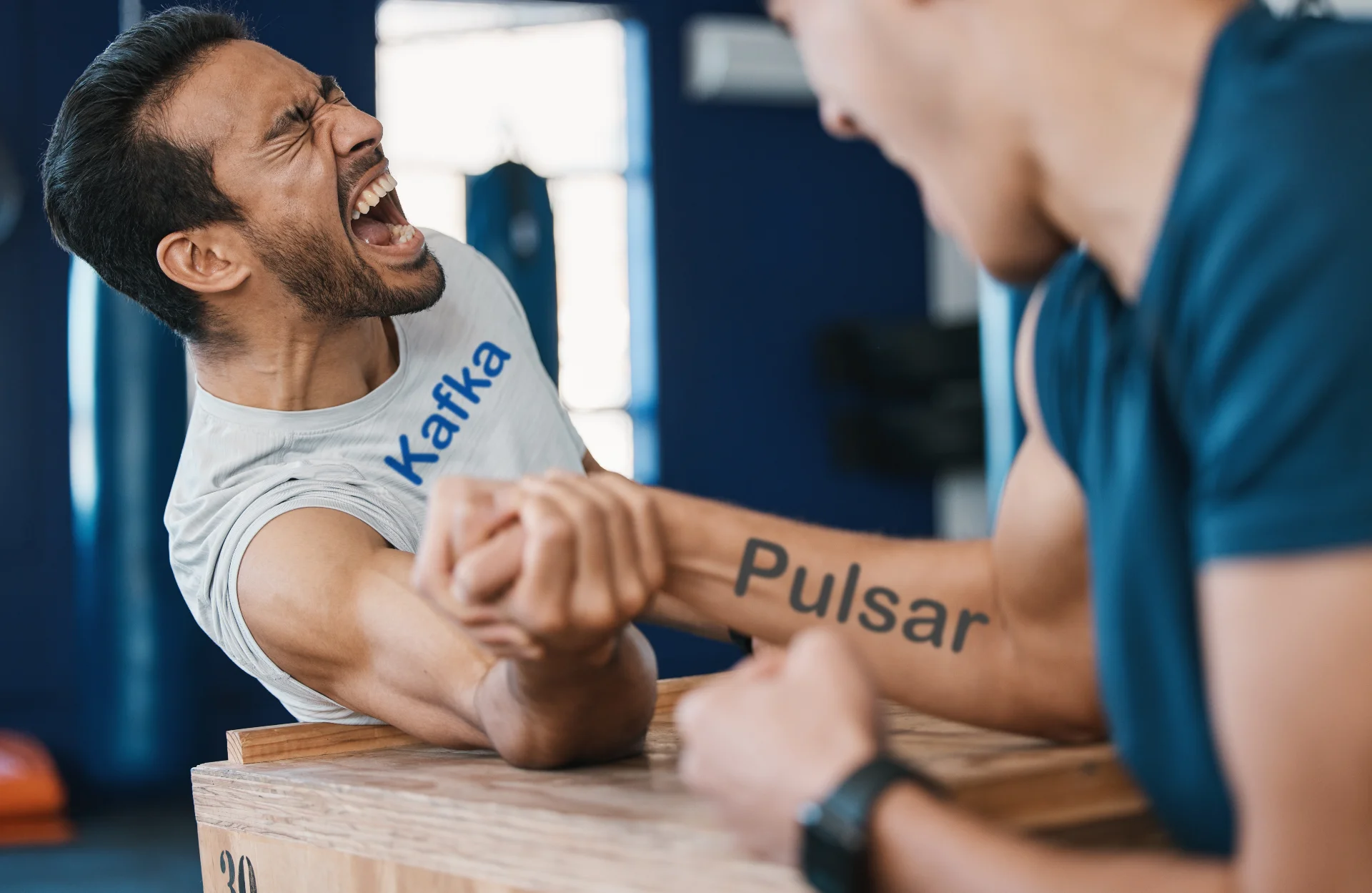
Ready to experience the new paradigm in data management?
Talk with our experienced team to bring unmatched efficiency and performance to your modern data center and data management processes.